As new antibody drug conjugates are developed, the question of which specific patients will respond to these therapies remains. This work develops a spatial PK/PD model for the antibody drug conjugate trastuzumab emtansine, T-DM1, and explores the relationship between HER2 (target) expression and other individual features of the tumor microenvironment using a computational approach.
SimBioSys (SBS) developed TumorSight for individualized treatment planning, coordinated care, and shared decision-making. TumorSight rapidly builds 3D computational models from standard breast MRI data.
Methods
We developed a spatial PK/PD model for T-DM1 based upon an allometrically-scaled mouse model (Jumbe, Nelson L., et al., 2010) and the reported outcomes from the KRISTINE trial, which investigated T-DM1 plus pertuzumab in the neoadjuvant early-stage breast cancer setting (Hurvitz, Sara A., et al., 2018). The T-DM1 spatial PK/PD model was then integrated into our previously described 4D biophysical simulation model (Howard, Frederick M., et al., 2022). The biophysical model leverages baseline breast MRI and clinicopathologic features and predicts the individual response to a treatment regimen.
Using digital twin tumors from our TumorBank to simulate the KRISTINE trial cohort (n=40), the PD model was parameterized to achieve the trial response rate. To orthogonally validate the spatial PK/PD model, volumetric response and pCR were predicted in an independent cohort of patients (n=44) who received T-DM1 followed by dose-dense doxorubicin and cyclophosphamide.
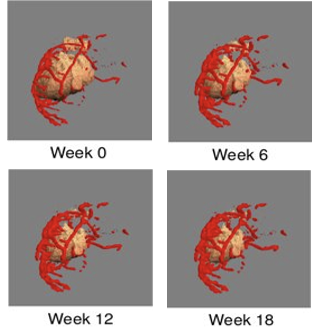
Figure 1. A spatial PK/PD model simulation demonstrating one tumor’s response to therapy over time.
We then assessed the added value of the target expression data by developing logistic regression models to predict which patients would achieve pCR based upon HER2 expression alone or HER2 expression combined with the final tumor volume simulated using the spatial PK/PD model.
Results
The spatial PK/PD model is independently predictive of achieving pathological complete response (pCR) as shown in Figure 2. Two of the spatial features which influence the response rate are the tumor metabolic activity and the spatial architecture of the tumor. These features are correlated with the rate of pCR as shown in Figure 3.
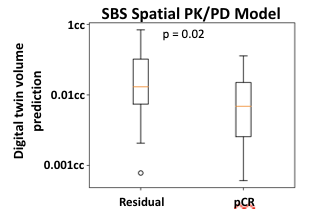
Figure 2. The predicted final volume from the spatial PK/PD model can classify which tumors will achieve pCR.
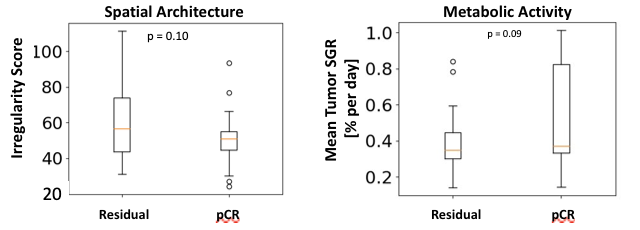
Figure 3. The predictive power of spatial features derived from TumorScope T-DM1 model simulations in classifying the rate of pCR.
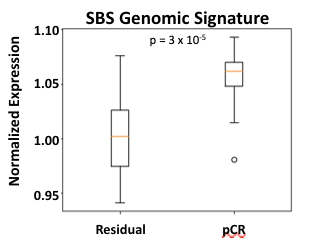
Figure 4. The SBS Genomic Signature represents individual HER2 overexpression as measured from transcriptomic data is predictive for the response to T-DM1-ddAC.
A logistic regression model based upon HER2 expression from transcriptomic data, the SBS Genomic Signature, is a strong predictor of response to T-DM1 (Figure 4). This model achieves an odds ratio of 9.6 and an area under the ROC curve (AUC) of 0.81 (Table 1).
The SBS tumorHER2 model is a logistic regression model which combined the Spatial PK/PD model with the genomic signature is superior to either model alone.
Results
The tumorHER2 model, which combines the HER2 expression with the spatial PK/PD model, improves the predictive value of the model with an odds ratio of 34.0 and an AUC of 0.88.
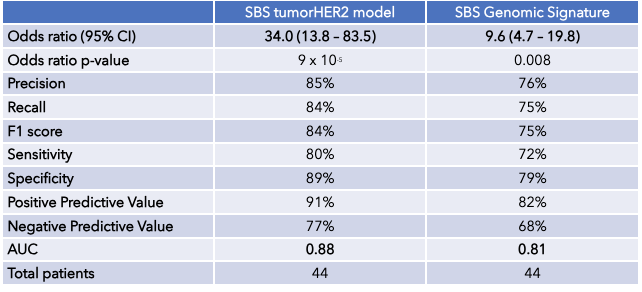
Table 1. Comparing the Predictive Capability of the SBS tumorHER2 model and the SBS Genomic Signature.
Conclusions
The spatial PK/PD improves prognostic predictions compared to HER2 gene expression data alone. In leveraging the SBS biophysical simulation platform, the spatial PK/PD model captures the individual variability in tumor growth rate, tumor perfusion, and drug disposition, all of which influence the treatment efficacy. The rate of target expression will highly influence response to an ADC, but the biophysical model demonstrates that features of the spatial tumor microenvironment also influence response.
References
- Jumbe, Nelson L., et al. “Modeling the efficacy of trastuzumab-DM1, an antibody drug conjugate, in mice.” Journal of pharmacokinetics and pharmacodynamics 37 (2010): 221-242.
- Hurvitz, Sara A., et al. “Neoadjuvant trastuzumab, pertuzumab, and chemotherapy versus trastuzumab emtansine plus pertuzumab in patients with HER2-positive breast cancer (KRISTINE): a randomised, open-label, multicentre, phase 3 trial.” The Lancet Oncology 19.1 (2018): 115-126.
- Howard, Frederick M., et al. “Highly accurate response prediction in high-risk early breast cancer patients using a biophysical simulation platform.” Breast Cancer Research and Treatment 196.1 (2022): 57-66.
About Us
SimBioSys is a software company deploying a combination of biophysical modeling and artificial intelligence to revolutionize precision cancer care. Our portfolio of software applications enables individualized treatment planning, accelerated drug development, clinical trial optimization and comprehensive biomarker development.
