Neoadjuvant therapy (NAT) has become a standard-of-care (SOC) for patients diagnosed with locally advanced or early-stage breast cancer. While pathological complete response (pCR) is commonly used to measure NAT effectiveness, patients who do not achieve pCR may still benefit from NAT, depending on the tumor’s response. A reduction in tumor extent could alter the surgical approach from mastectomy to breast conserving surgery and improve patient outcomes. In this study, we used pre-treatment imaging data and quantification from the TumorSight platform to provide a comprehensive prediction of clinically relevant metrics including tumor volume, surgical excision volume, and spatially derived metrics; this research represents an important advancement in predicting NAT effectiveness.
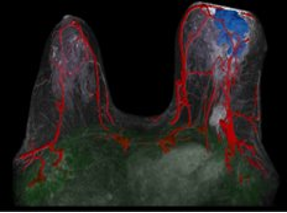
Figure 1. Automatic tissue segmentation, calculation of tumor volume, and calculation of spatial metrics within the TumorSight platform.
Methods
262 breast cancer patients from 5 institutions with pre- and post-NAT DCE-MRI were included in this study. Each MRI was automatically segmented using a convolutional neural network with manual edits and verification. Radiomic features were extracted from the pre-NAT MRI and tumor segmentation. The selected features were inputted into a Huber loss regression model to predict either the post-NAT tumor volume or the surgical excision volume. To enable spatial and morphological comparison between the predicted and post-NAT tumor, an erosion model was developed in which the pre-NAT and post-NAT MRIs were co-registered, and the pre-NAT tumor was symmetrically eroded until its volume matched the predicted volume. Clinically relevant metrics such as the distance from the tumor to the nipple, skin, and chest were computed to assess the morphological and spatial prediction capabilities of the model. All models were validated on patients from cohort holdout sets (n=110) unseen during training.
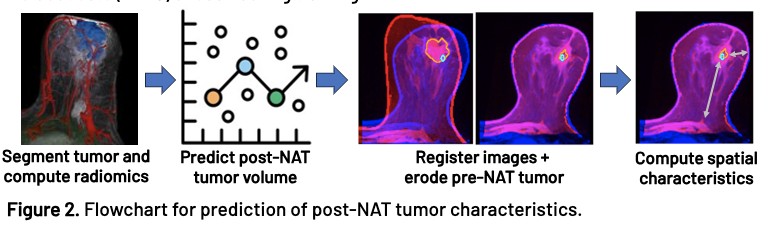
Results
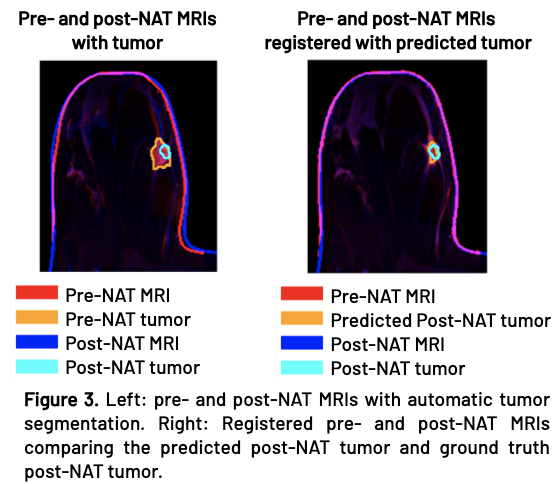
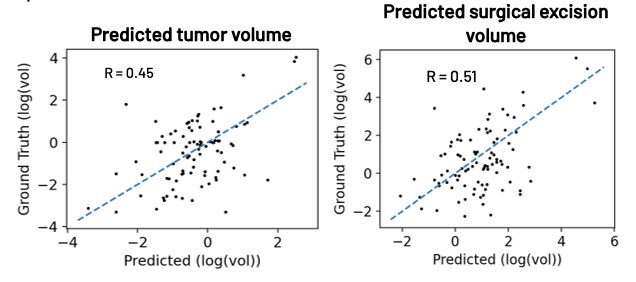
Figure 4. Prediction results for post-NAT tumor volume (left) and tumor excision volume (right) compared with ground truths. The blue dashed line shows an ideal prediction.
Following post-NAT volume prediction, erosion, and registration, the predicted and actual tumors align well (Figure 3). Figure 4 demonstrates good agreement between the predicted and ground truth post-NAT tumor and surgical excision volumes. The average volume errors for post-NAT tumor and excision volume predictions were 0.87 and 1.0 cc’s, respectively.
Results
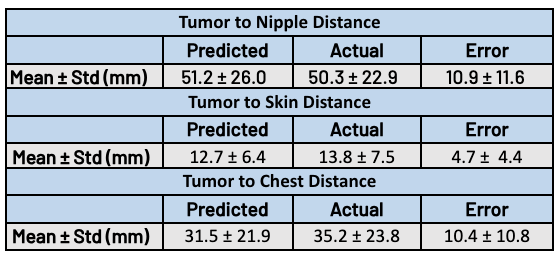
Table 1. Predicted vs. post-NAT tumor comparison. Results demonstrate accurate predictions for clinically relevant distance metrics including distance from tumor to skin, nipple, and chest.
Following tumor erosion, clinically-relevant metrics for surgical planning were computed, including distance from tumor to skin, nipple, and chest. These metrics are automatically computed as part of the TumorSight platform. Table 1 demonstrates close agreement between the predicted and ground truth spatial metrics.
Conclusions
This study demonstrates a successful approach towards predicting post-NAT tumor volume, surgical excision volume, and morphological and spatial characteristics. By providing reliable estimates of post-NAT tumor characteristics using SOC pre-NAT data, our predictive models enable personalized treatment planning and patient stratification to optimize patient care.