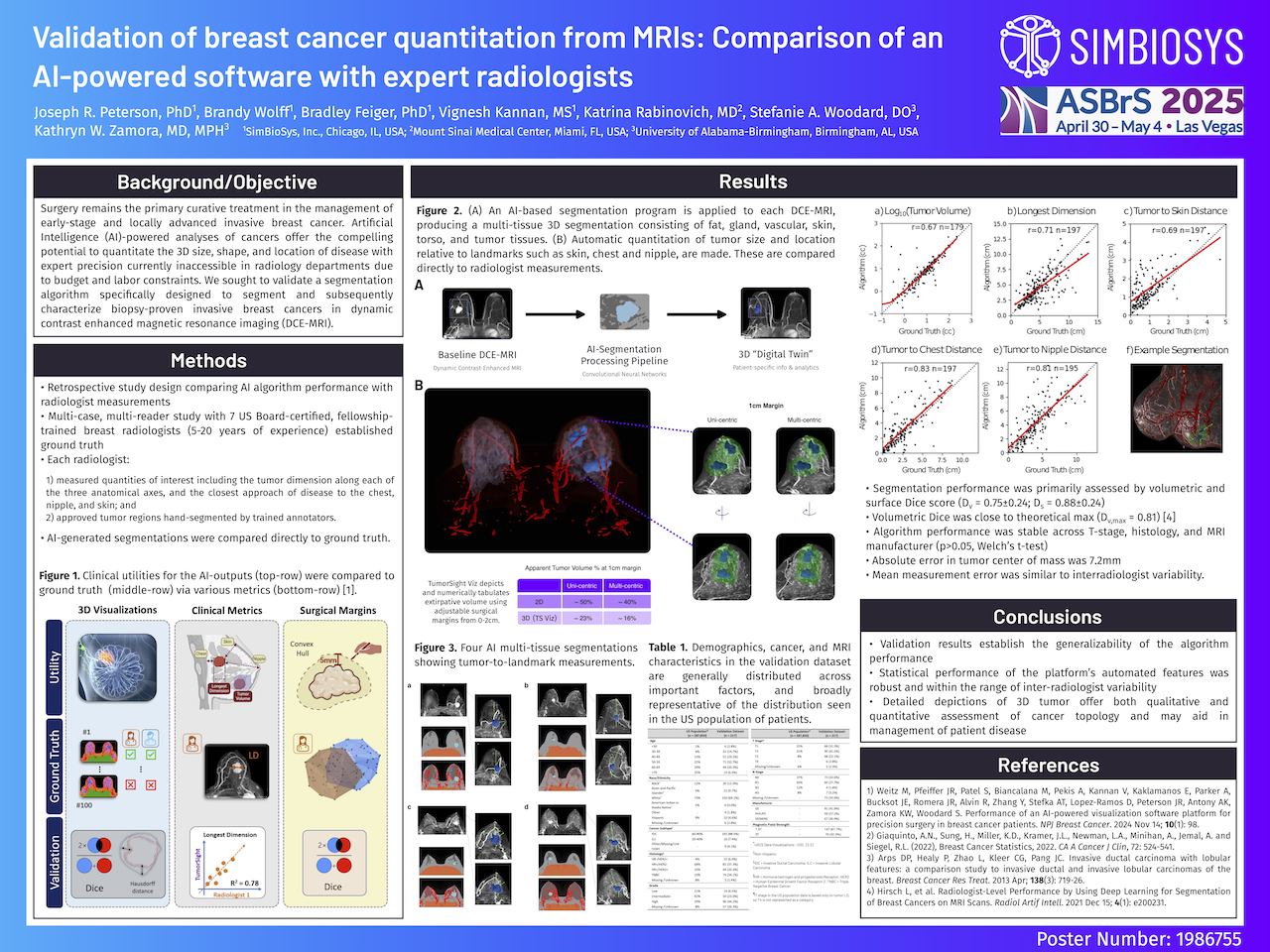
Authors: Joseph R. Peterson, PhD1, Brandy Wolff1, Bradley Feiger, PhD1, Vignesh Kannan, MS1, Katrina Rabinovich, MD2, Stefanie A. Woodard, DO3, Kathryn W. Zamora, MD, MPH3
1SimBioSys, Inc., Chicago, IL, USA; 2Mount Sinai Medical Center, Miami, FL, USA; 3University of Alabama-Birmingham, Birmingham, AL, USA
Background/Objective: Surgery remains the primary curative treatment in the management of early-stage and locally advanced invasive breast cancer. Artificial Intelligence (AI)-powered analyses of cancers offer the compelling potential to quantitate the 3D size, shape, and location of disease with expert precision currently inaccessible in radiology departments due to budget and labor constraints. We sought to validate a segmentation algorithm specifically designed to segment and subsequently characterize biopsy-proven invasive breast cancers in dynamic contrast enhanced magnetic resonance imaging (DCE-MRI).
Methods: A retrospective study assessing algorithm performance with ground-truth established by 7 US Board-certified, Fellowship-trained breast radiologists (5-20 years of experience) was conducted. Briefly, ground truth was established via a multi-case, multi-reader study where radiologists: 1) measured quantities of interest including the tumor dimension along each of the three anatomical axes, and the closest approach of disease to the chest, nipple, and skin; and 2) approved tumor regions hand-segmented by trained annotators. Algorithm-generated segmentations were compared directly to ground truth.
Results: Data from over 15 institutions geographically distributed throughout the US representing both Academic and Community practice settings were used to train or validate the device performance. The algorithm was trained on 676 patients, and subsequently validated with 202 patients.
Segmentation performance was primarily assessed by volumetric and surface Dice score, which quantify the similarity of the overall tumor segmentation (Dv) and the similarity of tumor boundary (Ds), respectively. An average Dv = 0.75±0.24 observed in the validation dataset was near that reported in studies examining interadiologist agreement where the median volumetric dice was found to be 0.81 (Hirsch et al. Radiology AI, 2021). Similarly, average surface dice, Ds = 0.88±0.24, was near the theoretical maximum (Ds,max = 1). Absolute error in tumor center of mass was found to be 7.2mm. Notably, this is across tumors of all sizes, and accounts for errors in both large and small tumors.
Tumor quantities of interest including tumor to landmark distances, and tumor volume were compared directly to radiologist measurements (see Figure). Statistically significant (p<0.01; t-test), and numerically substantial correlation was observed for tumor volume (r=0.67), longest dimension (r=0.71), tumor to skin distance (r=0.69), tumor to chest distance (r=0.83). and tumor to nipple distance (r=0.81). Additionally, mean distance error for each measurement was similar to observed interradiologist variability.
Finally, algorithm performance was found to be stable across clinical and imaging substrata. No statistically different segmentation performance (p>0.05, Welch’s t-test) was observed across T stage (T1-T4), histology (HR+/HER2-, HR+/HER2+, HR-/HER2+, TNBC), MRI manufacturer (GE, Siemens, Philips), or magnetic field strength (1.5T, 3T).
Conclusions: Because data from each institution was used solely to train or to validate the algorithm, the validation result establish the generalizability of the algorithm performance. The statistical performance of the platform’s automated features was robust and within the range of inter-radiologist variability. These detailed depictions of 3D tumor offer both qualitative and quantitative assessment of cancer topology and may aid in management of patient disease.